Control of Genotoxic and Elemental Impurities
(Q)SAR Systems
A (Q)SAR (Quantitative structure–activity relationship) model can estimate the relationship between the structure of chemical substances and another property, e.g. the substance’s ability to cause toxic effects.
(Q)SAR models can therefore be used to predict the properties of substances that are harmful to the environment or health.
For Phase 2b and Phase 3 clinical development trials, a list of the impurities assessed by (Q)SAR should be included, and any Class 1, 2, or 3 actual and potential impurities should be described along with plans for control.
The in silico (Q)SAR systems used to perform the assessments should be described. The results of bacterial mutagenicity tests of actual impurities should be reported.
AMES/QSAR International Collaborative Study
Robust QSAR models defining toxicological endpoints are desirable to enable regulatory authorities to identify chemicals possibly causing adverse effects without performing actual toxicological studies. Much effort has been invested in the development of QSAR models to predict Ames mutagenicity, among many toxicological endpoints, to exploit the large body of Ames data and the strong correlation between chemical structure and Ames mutagenicity. Ames results are important for decisions on the development of chemical products and pharmaceuticals and the assessment of chemical safety, given that a positive result corresponds to increased cancer risk from exposure to the chemical even at a low level.
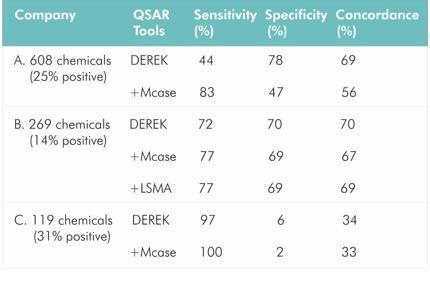
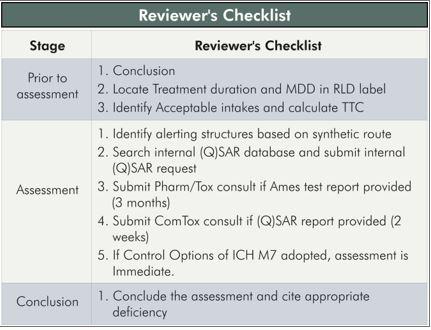
The ICH-M7 guideline currently recommends two QSAR models (expert rule-based and statistical) to predict Ames mutagenicity for initially assessing DNA-reactive impurities in pharmaceuticals. This is the first international guideline addressing the use of QSAR in lieu of an actual toxicological study for human health assessment. Thus, QSAR models for Ames mutagenicity now require much greater prediction power to ensure the safety of chemicals. To increase this prediction power, experimental data sets as training data to build the models are important. Large numbers of highly reliable data sets will allow development and improvement of QSAR models with high predictive power.
The Division of Genetics and Mutagenesis, National Institute of Health Sciences (DGM/NIHS) has Ames mutagenicity data for approximately 12,000 new chemicals. The Ames assays were conducted according to the OECD TG471 guideline and Industrial Safety and Health Act in Japan under GLP-compliant conditions. DGM/NIHS now provides these Ames data to QSAR builders/vendors to improve their QSAR models for predicting Ames mutagenicity with the permission of the Industrial Safety and Health Department of the Ministry of Health, Labor and Welfare (MHLW), Japan. The Ames/QSAR international collaborative study leaded by DGM/NIHS was launched on 2014. Because most of the Ames data are confidential, the QSAR builders/vendors participating in the project must execute a confidentiality agreement. Twelve QSAR builders/vendors from USA, UK, Italy, Spain, Bulgaria, Sweden, and Japan are currently participating in this project.
Chemical Classes in Industrial Chemicals (CPPB) and Expected Chemicals as impurities
Industrial Chemicals: Aromatic amine or amide, N=Nitro or N-nitroso compound, Aromatic nitro compound, Alkylating agent, Aromatic azo compound, Epoxide, Halogenated alkene, Misc Alerts (<5).
Expected Chemicals as impurities: Alkylating agent, Aromatic amines, amides, and N-hydroxy-lamines, Aromatic nitro derivatives, Acid chloride derivatives, Alkyl aldehydes, Hydrazine derivatives, Boronic acids, Misc Alerts (<20).